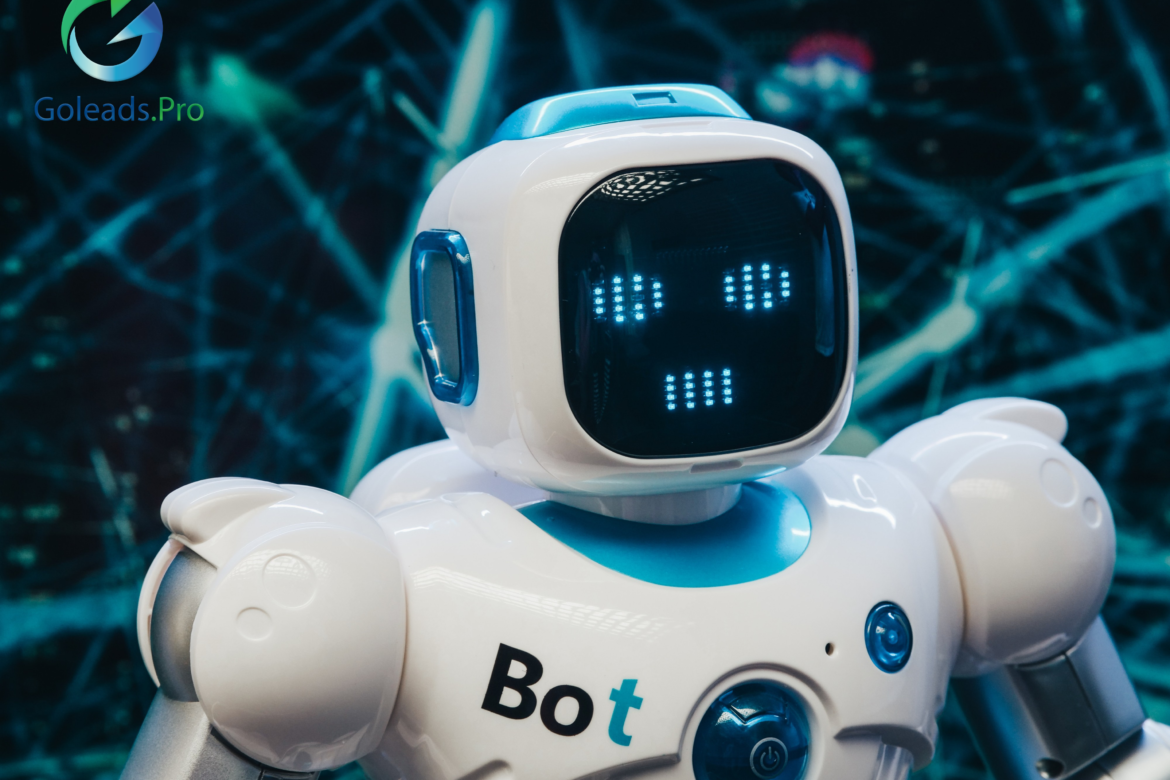
Welcome esteemed readers, to this scholarly exploration of Large Language Models (LLMs) and their remarkable insurgence in Artificial Intelligence. As a Harvard University Professor, I am honoured to present this comprehensive blog, enriched by the insights of Mr. Suman Sarkar, the distinguished Owner and CEO of Goleads.pro, with his decade-long expertise in the software industry and profound involvement in AI & LLM. Together, we embark on an intellectual journey to unravel the profound impact of LLMs, with a particular focus on the revolutionary Chat GPT (Generative Pre-trained Transformer) and Google Bard.
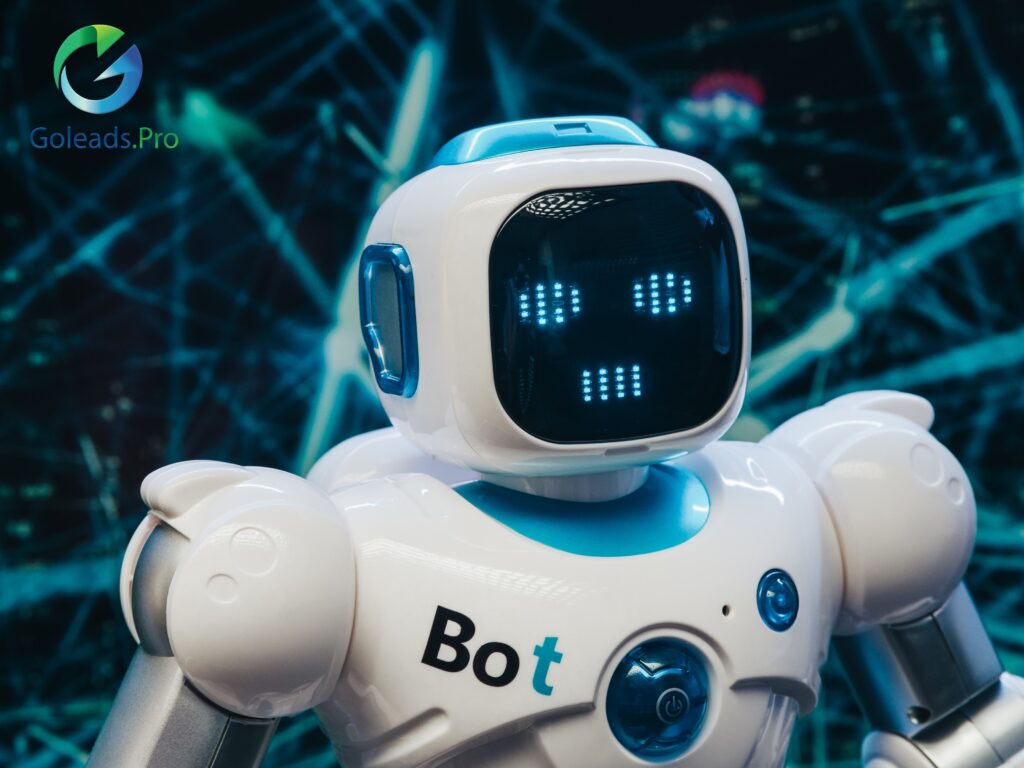
I. Unraveling Large Language Models (LLMs):
Large Language Models, characterized by their vast parameter size and deep neural network architectures, have become prominent in contemporary AI research and applications. These models excel in understanding, generating, and processing human language, making them indispensable across industries, including customer service, content generation, and research.
– LLMs: A Definition and Purpose:
LLMs, also known as AI language models, are sophisticated machine learning systems that leverage natural language processing (NLP) techniques and neural networks to process and generate human-like text.
– The Role of AI in LLM Development:
Artificial Intelligence plays a central role in training and fine-tuning LLMs. The models learn from vast datasets to grasp language patterns, and their subsequent fine-tuning tailors their knowledge to domain-specific tasks.
– LLM Applications in the Software Industry:
LLMs have been integrated into various software applications, transforming how businesses interact with customers, generate content, and streamline operations.
II. The Groundbreaking Emergence of GPT:
A. GPT’s Historical Evolution:
– The Inception of GPT-1 and Its Early Significance:
In 2018, OpenAI unveiled GPT-1, featuring 117 million parameters. It demonstrated impressive language generation but lacked depth of understanding.
– The Leap to GPT-2: Breaking New Frontiers:
In 2019, GPT-2 with 1.5 billion parameters surpassed its predecessor, demonstrating a substantial improvement in coherent text generation, raising concerns about potential misuse.
– GPT-3: A Milestone in AI-Language Understanding:
Released in 2020 with a staggering 175 billion parameters, GPT-3 exhibited unprecedented language understanding and the ability to perform a wide range of tasks with minimal fine-tuning.
B. The Working Principles of GPT:
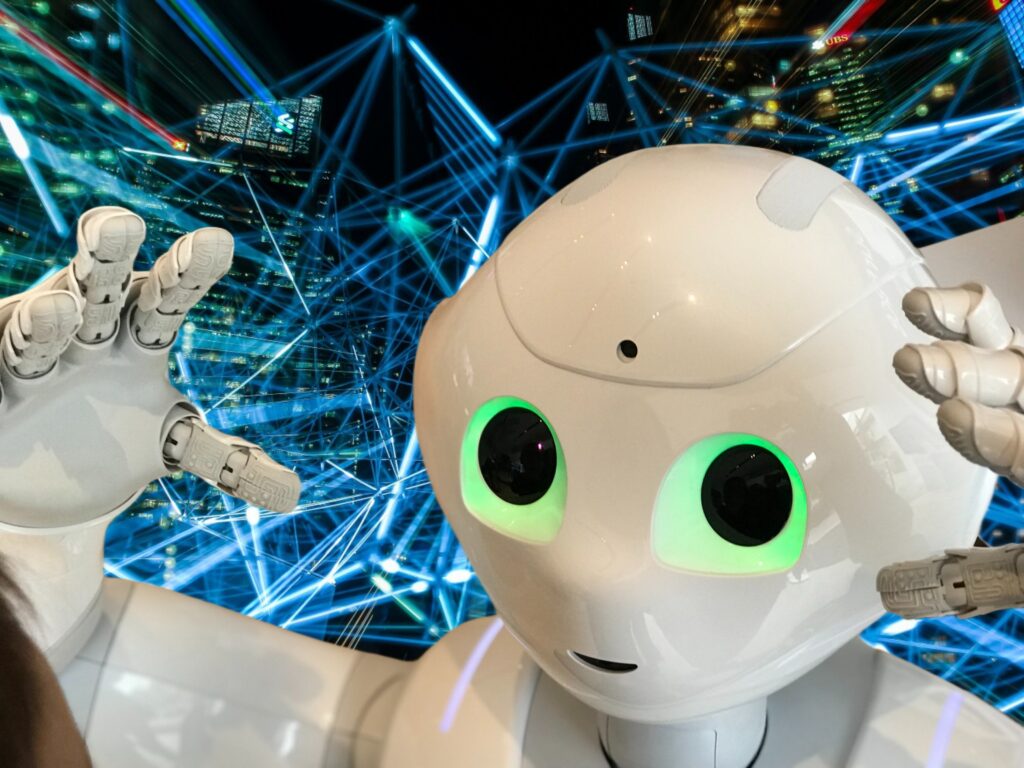
– A Transformer-based Architecture:
GPT’s underlying transformer architecture, with self-attention mechanisms, empowers it to process extensive contextual information and generate coherent responses.
– Unveiling the Power of Pre-training and Fine-tuning:
Pre-training on a diverse dataset enables GPT to grasp general language patterns, while fine-tuning tailors its knowledge to specific tasks, contributing to its versatility.
Benefits of Chat GPT | Limitations of Chat GPT |
---|---|
1. Natural Language Understanding: Chat GPT exhibits exceptional language comprehension, allowing it to understand and generate human-like responses. | 1. Lack of Context Sensitivity: While Chat GPT can generate coherent text, it may struggle with context-sensitive responses, leading to occasional inaccuracies or inappropriate answers. |
2. Versatility: Chat GPT can be fine-tuned for various tasks, making it adaptable across different domains, including customer service, education, and creative writing. | 2. Bias Amplification: The model’s training data may contain inherent biases, leading to biased or discriminatory responses, reflecting societal prejudices present in the data. Careful handling is required to minimize such biases. |
3. Time and Cost Efficiency: Implementing Chat GPT can save time and resources in handling customer queries and providing support, thereby improving overall operational efficiency. | 3. Ethical Concerns: Chat GPT may inadvertently generate misleading information or misinformation, necessitating robust safeguards to avoid spreading false content. |
4. Scalability: Chat GPT can handle multiple concurrent conversations, enabling it to cater to a large number of users simultaneously. | 4. Sensitivity to Input Phrasing: Chat GPT’s responses can vary depending on slight changes in input phrasing, leading to inconsistent results. This requires careful monitoring to maintain response coherence. |
5. Multilingual Capabilities: Chat GPT can be fine-tuned to support multiple languages, facilitating global accessibility and communication. | 5. Lack of Empathy: As an AI model, Chat GPT lacks genuine emotional understanding, potentially providing responses that seem cold or detached in emotionally sensitive situations. |
6. Continuous Learning: Chat GPT can be continuously updated and improved based on user feedback, ensuring a dynamic and evolving conversational experience. | 6. Over-Reliance on Training Data: Chat GPT’s responses heavily depend on the quality and diversity of its training data. Inadequate or biased training data can hinder its performance. |
7. Data Privacy Concerns: Chat GPT may handle sensitive user data during conversations, necessitating robust data privacy measures to safeguard user information. | 8. Turing Test Challenges: While Chat GPT excels in natural language understanding, it may still struggle to pass the Turing Test consistently, making it challenging to fully replicate human-like conversations. |
9. Limited Common Sense Reasoning: Chat GPT may not possess the ability to infer certain common sense knowledge, leading to an occasional lack of logical reasoning in responses. |
III. Google Bard: An Innovative Contender in the LLM Arena:
A. Introducing Google Bard:
– A Response to GPT’s Dominance:
Google Bard emerges as a specialized LLM designed to cater to the legal domain, offering enhanced capabilities in handling legal texts and contexts.
– The Quest for Specialization in Legal Language Processing:
Google Bard’s architecture emphasizes long-range context retention and coherence, making it ideal for complex legal documents and research.
B. Comparative Analysis of GPT and Google Bard:
– Parameters, Performance, and Domain Expertise:
Both GPT and Google Bard leverage transformer-based architectures, but the latter focuses on optimizing legal language processing. Google Bard’s contextual retention empowers it to excel in the legal domain.
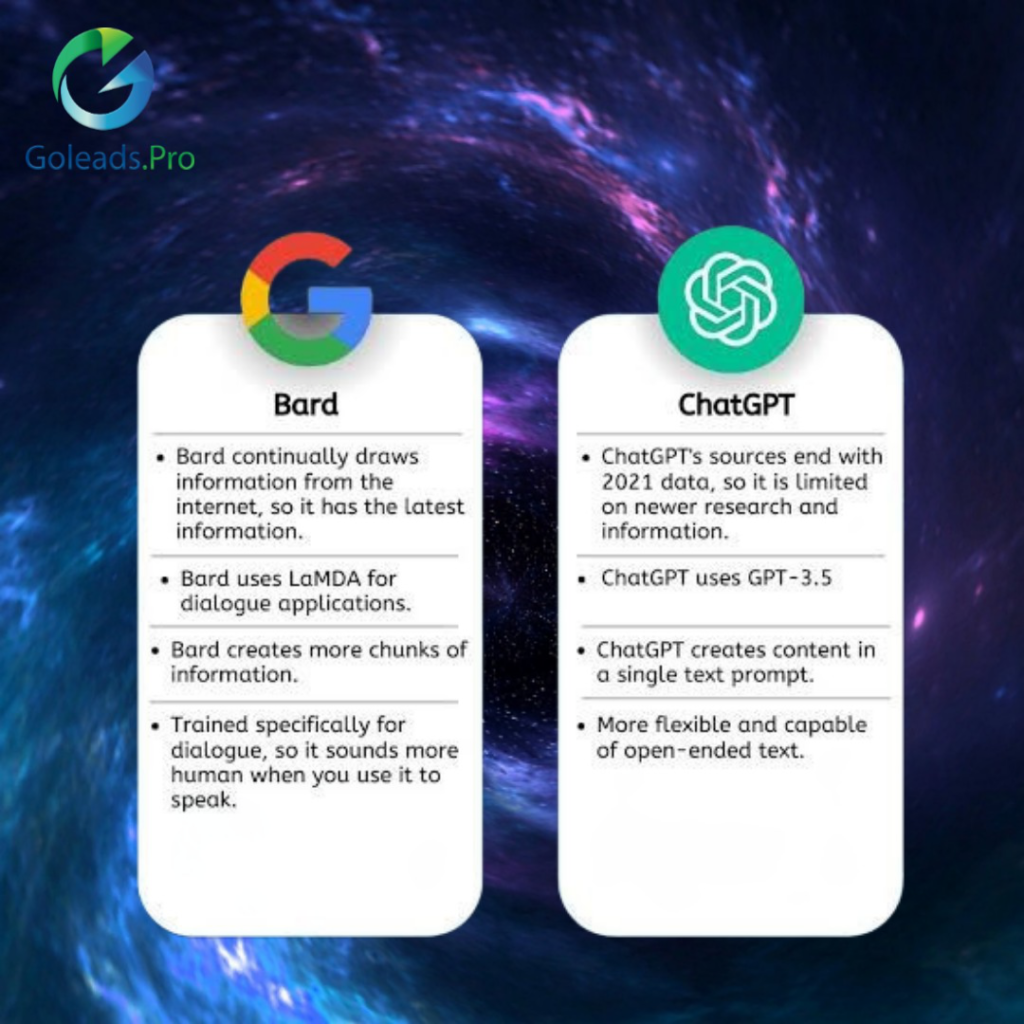
IV. LLM Technology Adoption: Trends and Statistics:
A. Table: The Ascendance of AI in Select Countries:
Country | AI Technology Adoption (2019) | AI Technology Adoption (2023) | Percentage Increase |
---|---|---|---|
USA | 45% | 65% | 44.44% |
UK | 38% | 55% | 44.74% |
Australia | 30% | 50% | 66.67% |
Canada | 35% | 60% | 71.43% |
Table: An analysis of AI technology adoption in select countries, comparing the adoption percentage in 2019 and projected for 2023. The substantial increase in AI integration reflects the growing significance of LLMs in global industries.
V. Impact of GPT and Google Bard on the Software Industry:
A. Enhancing Human-Machine Interaction:
– From Language Understanding to Conversational AI:
GPT and Google Bard’s language comprehension enable more natural and human-like interactions, enriching the customer experience.
– The Profound Implications for Customer Service and Support:
AI-driven LLMs have revolutionized customer support, streamlining query resolution and reducing response times, ultimately leading to improved customer satisfaction.
B. Driving Innovations in Language-Driven Applications:
– Creative Writing, Content Generation, and Storytelling:
LLMs like GPT are driving innovations in creative writing, generating compelling content, and even co-authoring with human writers.
– Optimizing Content Marketing and SEO Strategies:
By automating content generation, LLMs enhance content marketing efforts and boost SEO, enabling businesses to reach wider audiences.
VI. Ethical Considerations and Challenges:
A. Navigating Bias and Fairness in LLMs:
– The Dilemma of Training Data Biases:
LLMs, trained on extensive datasets, may inadvertently inherit biases, requiring meticulous curation and bias mitigation strategies.
– Striving for Ethical AI Decision-Making:
Responsible deployment of LLMs necessitates transparency and accountability in AI decision-making processes.
B. Ensuring Transparency and Explainability:
– The Quest for Interpretable LLMs:
AI models like GPT and Google Bard must be engineered to provide human-readable explanations for their responses, fostering trust and understanding.
– Mitigating the “Black-Box” Conundrum:
Efforts to unlock the inner workings of LLMs enable researchers to identify and address potential risks and limitations.
VII. The Future of LLM Technology:
A. Evolving LLMs: Beyond GPT and Google Bard:
– Multimodal Language Models and Hybrid Architectures:
The future of LLMs lies in the exploration of multimodal models, combining text, images, and other data modalities for enhanced understanding.
– Encouraging Interdisciplinary Research and Collaborations:
Universities, industry, and governments must collaborate to address ethical, technical, and policy challenges related to LLMs.
B. Unlocking the Potential of LLMs:
– Opportunities in Legal, Healthcare, and Financial Sectors:
LLMs like Google Bard hold immense potential in revolutionizing legal research, healthcare data analysis, and financial risk assessment.
– AI Ethics
and Policy Implications:
Continued research and dialogue are essential in crafting robust AI ethics frameworks and policies to govern the responsible use of LLMs.
Conclusion:
As we draw the curtains on this enriching discourse, the impact of Large Language Models on the software industry is undeniable. From the revolutionary GPT to the specialized Google Bard, these LLMs are reshaping human-machine interactions, redefining content generation, and pushing the boundaries of AI language understanding.
As a Harvard University Professor, I commend Mr. Suman Sarkar’s dedication to AI and LLM in Goleads.pro, catering to clients across nations. The dynamic integration of GPT and Google Bard marks a transformative era for AI technology and beckons us to tread thoughtfully through the ethical landscape, ensuring the responsible deployment of LLMs for the betterment of society.
May this extensive blog ignite further curiosity and academic pursuit, inspiring students, scholars, and industry professionals to explore the boundless horizons of Large Language Models and their profound implications in the AI revolution. Let us continue our collective journey of discovery, unleashing the full potential of LLMs while upholding the highest ethical standards in their use.